Contents
The Basics
The Applications
The Coverage
The Differentiators
Example Use Case
The Basics
Using pure machine learning, Alexandria has constructed the first AI analyst. Its high-performance engine handles massive amounts of unstructured content and accurately identifies the hidden sentiment, critical facts, and key relationships buried within it. Alexandria extracts market sentiment from tens-of-thousands of daily financial news items almost instantly, which gives firms the ability to plug news into investment strategies as a predictive and orthogonal factor for alpha and risk.
The product information and content statistics contained in this document are as of March 2018.
The Applications
Use Case
|
Description
|
Quantitative Research
|
New source of alpha for quantitative investment models
|
Portfolio Management
|
Use as a timing tool to speed up or delay trades
|
Risk Management
|
Orthogonal risk factor
|
Market Making
|
Widen or narrow spreads based on news volume and sentiment
|
Asset Allocation
|
Overweight / underweight regions or sectors based on aggregated sentiment
|
Event Detection
|
Identify news related to FDA announcements, MNA, guidance
|
Discretionary Managers
|
Create screens for a given sector to identify desirable and undesirable companies based on news sentiment
|
Compliance
|
Monitor news for company holdings
|
The Coverage
The ACTA Equity Data Feed covers a global universe of public companies starting in January 2000 (Figure 1). As part of the Open:FactSet Marketplace, the security level data provided by Alexandria has been standardized and reconciled to FactSet Symbology. This process involves mapping companies within the ACTA universe to the relevant FactSet Regional Identifier at a given point in time.
Maintaining ACTA data at the regional level provides users with three key benefits. First, FactSet’s entity-centric symbology model connects entities to security and listing level identifiers across multiple asset classes. This means users can link ACTA sentiment values to any exchange or listing tracked by the FactSet Symbology Master. Second, by integrating data into the FactSet Symbology framework users can seamlessly blend ACTA news sentiment and any content available within the Open:FactSet Marketplace. Third, this model allows users to explore network efforts across entity level relationships, including a company’s supply chain or throughout its corporate hierarchy.
Figure 2 provides a breakout in coverage by source type. Alexandria categorizes content into 12 unique sources with Dow Jones Newswire having the largest volume of news.
Figure 1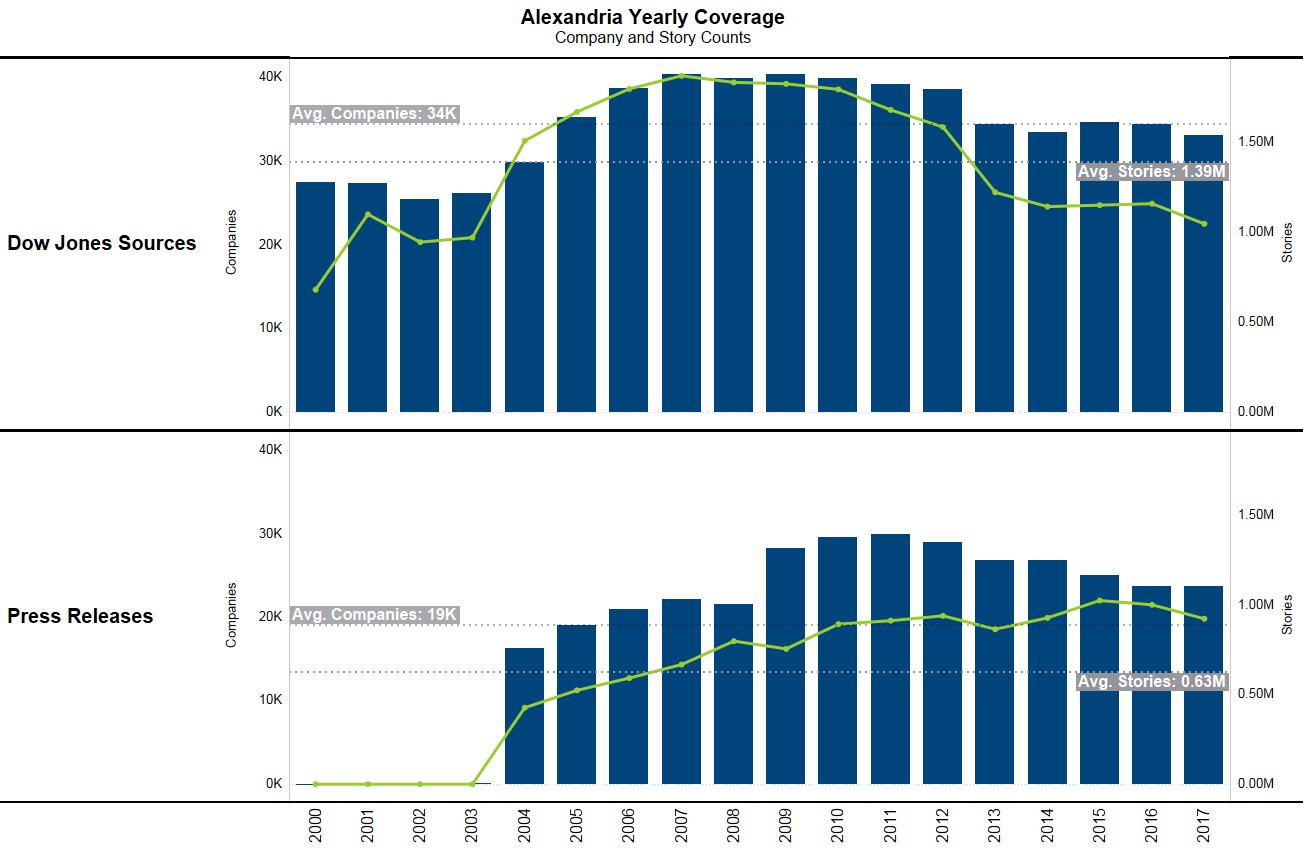
Figure 2
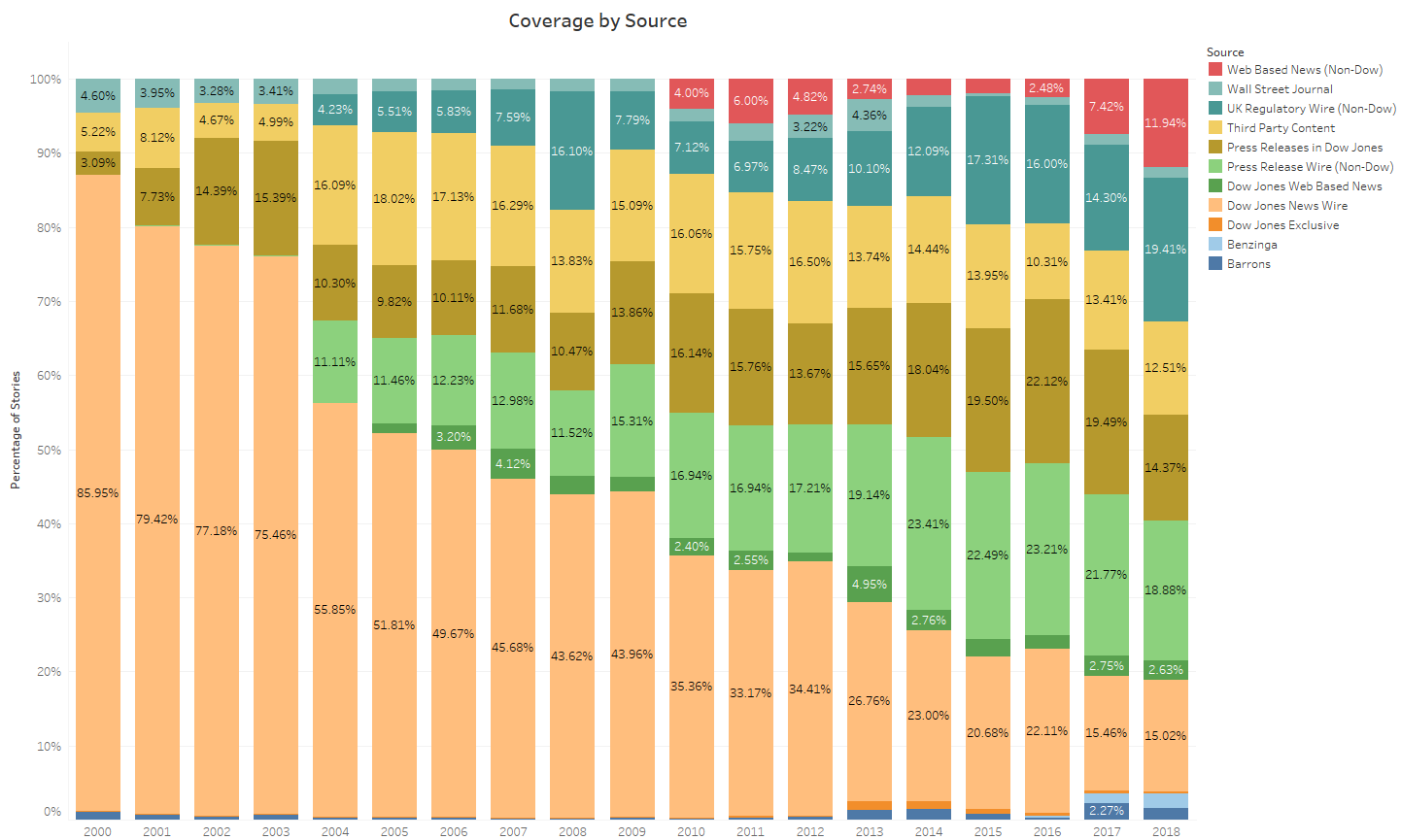
At the company level, Alexandria offers global coverage beginning in January 2000. Figure 3 provides a breakout of regional company coverage by year. The counts represent the number of unique companies with at least one story in the given year per region. Figure 4 provides a more detailed view of history by examining the top five countries in terms of unique companies covered within each region.
Figure 3
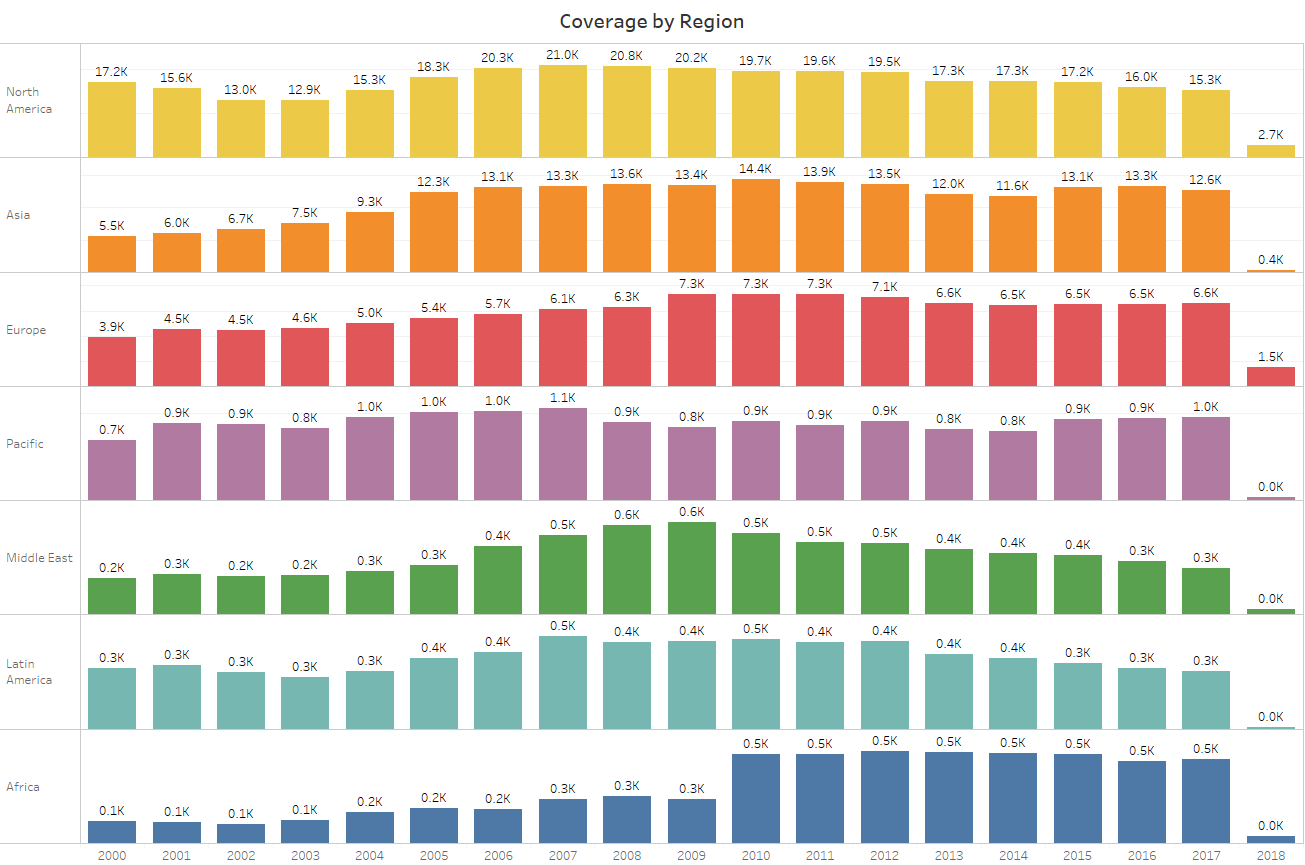
Figure 4
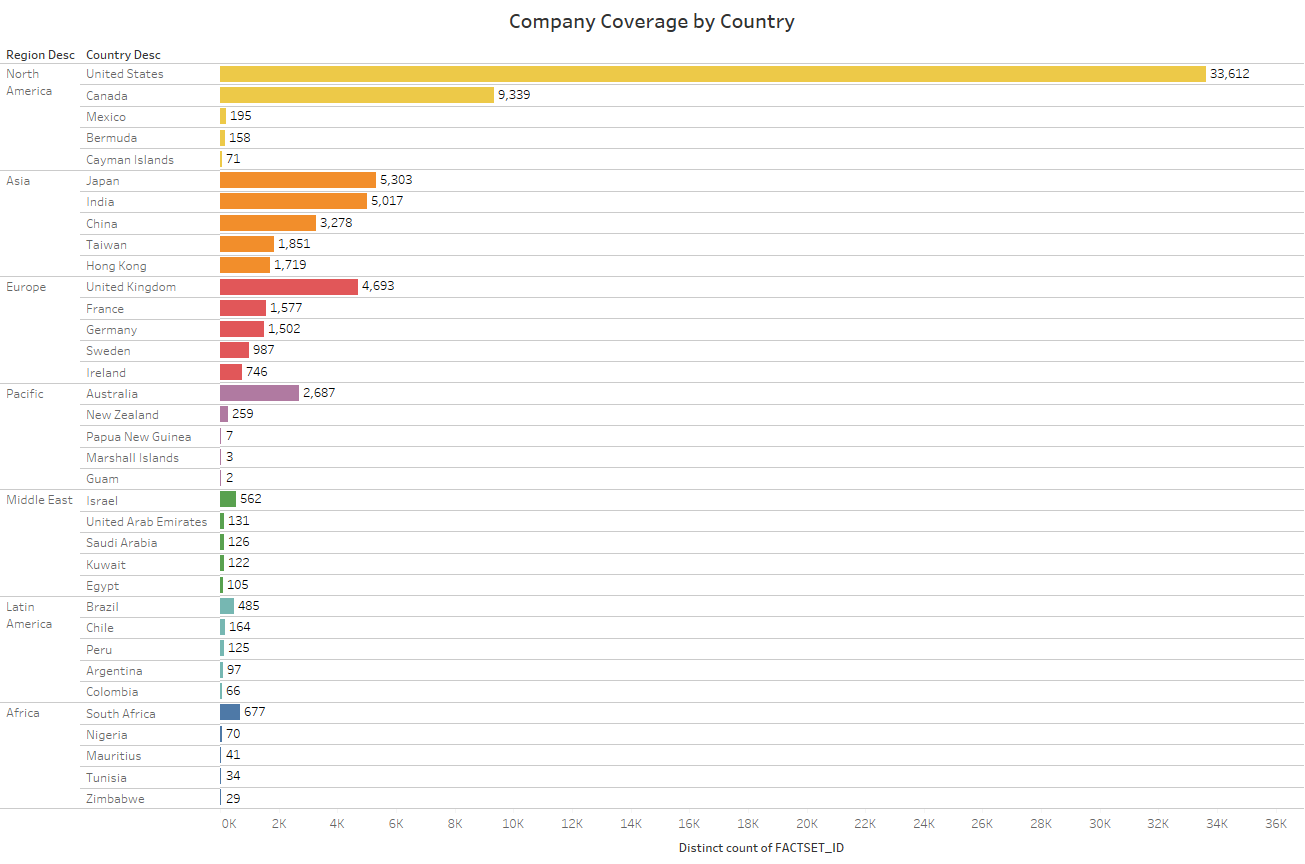
The Differentiators
Beyond coverage and history, key factors to consider when evaluating a news sentiment provider are the content analysis method, sources, classification/event tagging, and most importantly, entity identification.
Alexandria utilizes artificial intelligence algorithms to analyze sentiment, identify entities, and classify stories by event types. The algorithms are trained using historical new stories reviewed and annotated by buy-side research analysts. Leveraging this work, the data provided by Alexandria has been out of sample since 2005.
Research shows that an artificial intelligence or machine learning-based approach increases the accuracy of event classification and results in a higher rate of non-neutral stories compared to a dictionary or word-list approach. From our evaluation of its data, Alexandria is able to produce non-neutral sentiment scores for 38.5% of Dow Jones articles and 11.91% of press releases vs. a dictionary or rule-based sentiment calculation average of ~20%.
Alexandria analyzes and categorizes articles by event types in addition to sentiment analysis. Its taxonomy covers 31 event types including clinical trials, mergers, and acquisitions, and government interactions. Overall, 60% of Dow Jones and 53% of press releases stories are tagged with at least one event type. According to Alexandria, other sentiment providers achieve an average of ~10% event classification across all content. An increase of coverage for non-neutral stories combined with greater context through event tagging provides a more complete picture of the impacts news may have and insights into potential catalysts.
It is no surprise that earnings news is by far the most prevalent type within the data set, given its frequency for companies across the globe. More impactful is the large volume of stories that are tagged to market moving news events such as M&A, guidance, operations, and management changes.
Figure 5
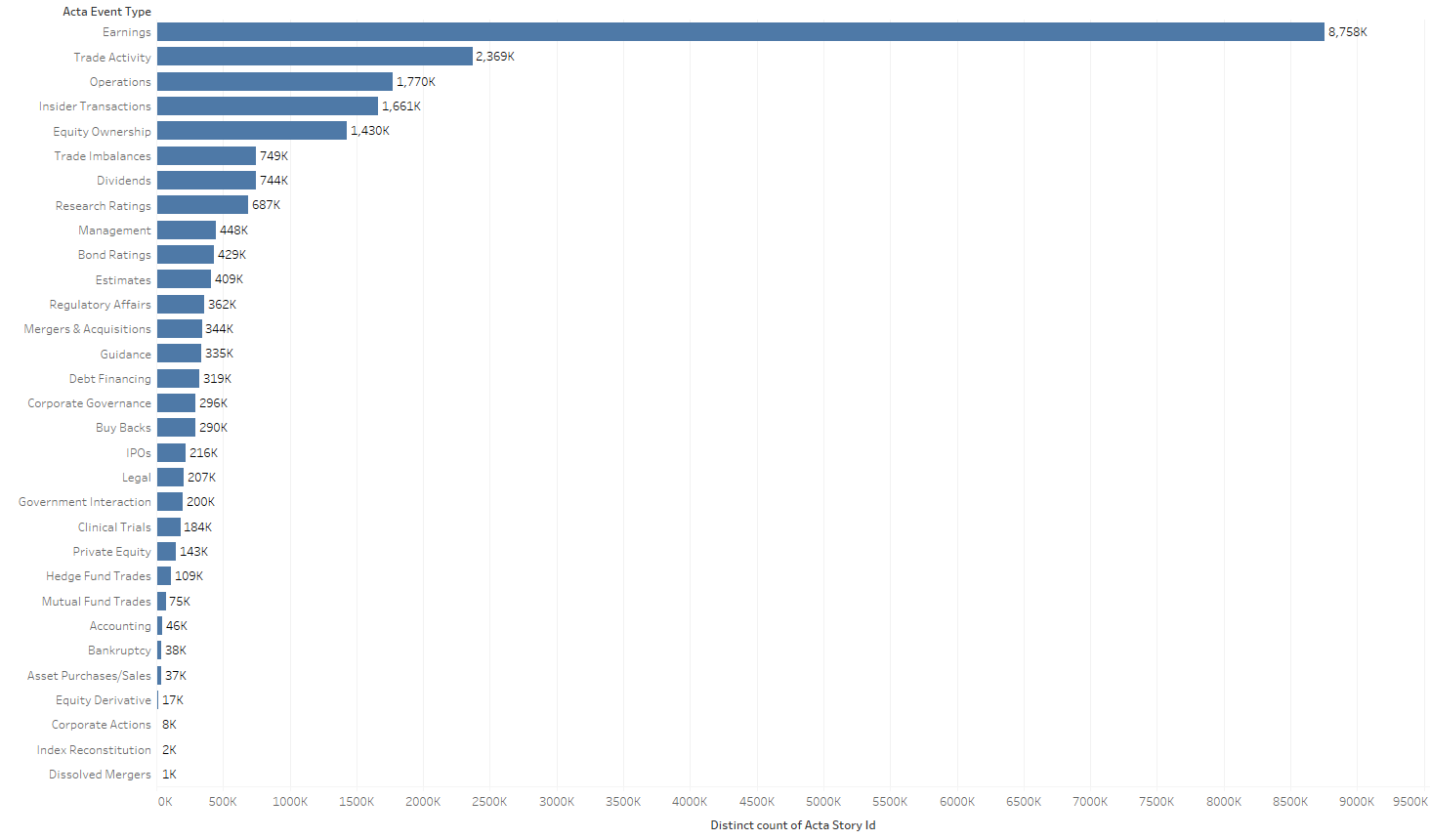
Example Use Case
A simple but effective application of the ACTA content is determining the predictive power of a daily sentiment value. To conduct this analysis, Alexandria’s sentiment data was extracted for the constituents of the S&P 1500 from January 1, 2000 to November 31, 2016.
While Alexandria provides several attributes associated with each story, this analysis filtered news based on Relevance. The Relevance field provides a weighting of the sentiment relative to the company identified within the news story. Relevance of ranges from 0 to 1, with 1 representing primary significance. For this analysis, only stories that held a Relevance of 1 were included.
The daily net sentiment is defined as the log((Positive Stories +1) / (Negative Stories+1)). A day is defined as stories published between 4:00 p.m. EST the previous day to 3:30 p.m. EST on the current day. In order to perform this aggregation, story date times must first be converted from UTC to EST.
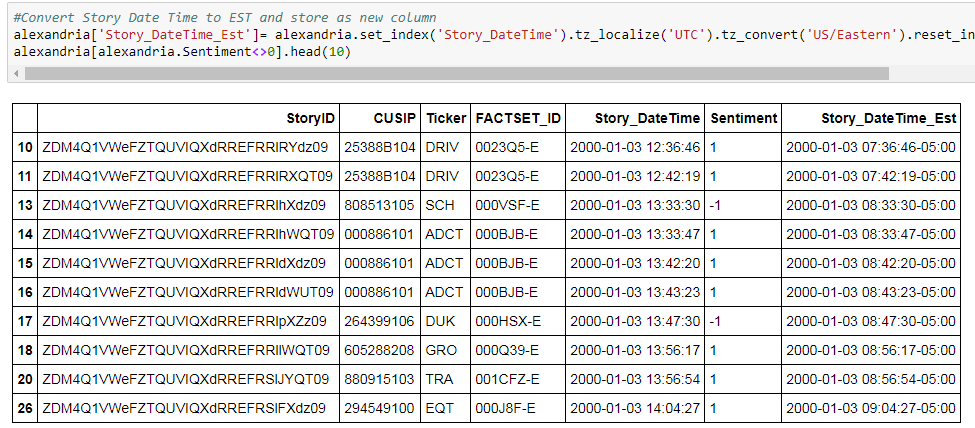
In order to simplify the aggregation of positive and negative stories over our defined daily window, stories published after 4:00 p.m. EST are assigned a story date of the next day:
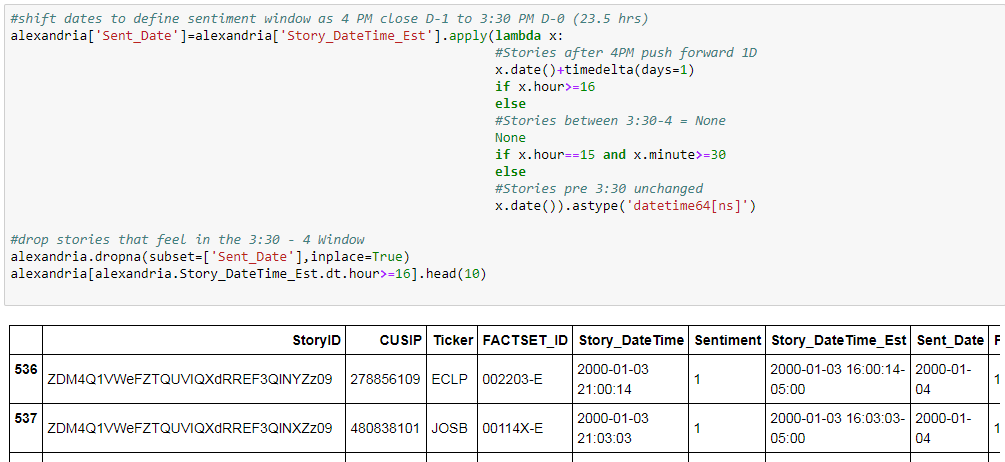
Finally, now that we have the correct date alignment we can calculate the daily net sentiment for each company:
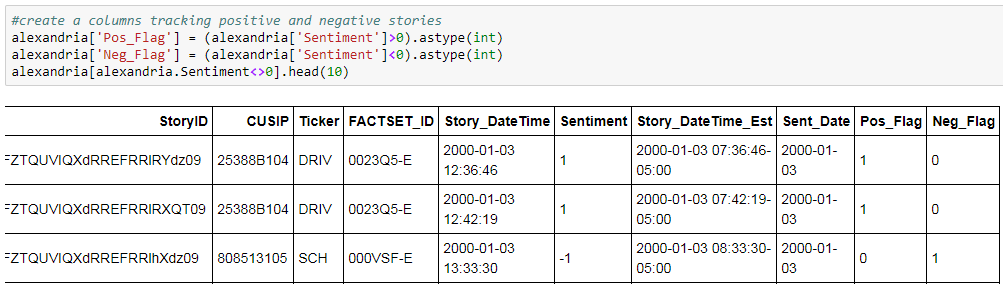
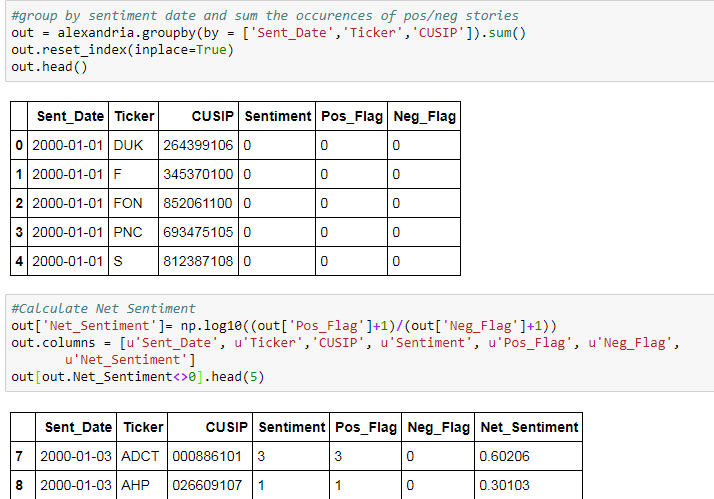
Companies were then ranked by sentiment and split into quantiles, with Q1 being the most negative and Q5 the most positive. To handle the abundance of no news and neutral companies on a given day, quintile breakpoints were established at -.45, -.1, .1, and .45. While this produced a limited universe in Q1 (31 companies per day on average) and Q5 (27 companies per day on average), both one and five day forward returns followed expectations with more positive news outperforming those with negative news.
Figure 6
Moving beyond aggregate sentiment, Alexandria’s robust and consistent event tagging facilitates thematic filtering of news. For example, deconstructing the average monthly sentiment values for Apple since the launch of the iPhone X highlights the variance across event types. The overall sentiment across all news for Apple was slightly negative at -.0441 in September 2017; however, news specific to Earnings (analysis, forecasts, analysts’ polls, and analyst estimates) was positive at .44. Filtering or weighting sentiment by event type gives investors yet another dial to turn in developing a company, industry, or time specific sentiment factor.
Figure 7 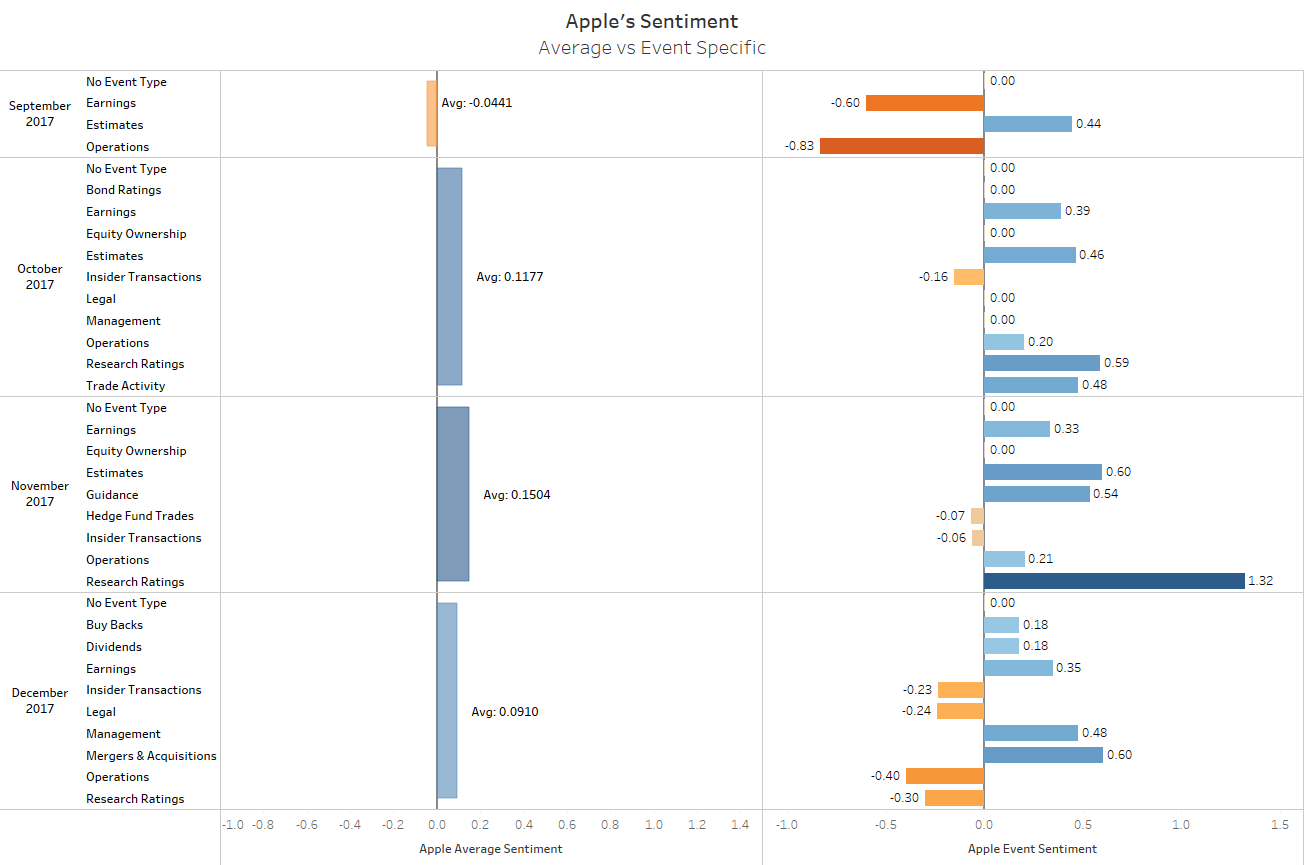
Network effects are more pronounced than ever in today’s global economy. A company’s success permeates up and down its supply chain. The effect is no different with news. Referring back to Apple, in the months and weeks leading up to the launch of a new product, stories often source information from the company's supply chain. Will delays in the production of new displays affect volume or delay shipping? Understanding the network effects of sentiment across a company’s supply chain allows for an examination of the impacts of news both upstream and downstream of a given firm. Since the launch of the iPhone X, for example, the sentiment of companies within Apple’s supply chain (green area) can lag or lead that of the Apple itself:
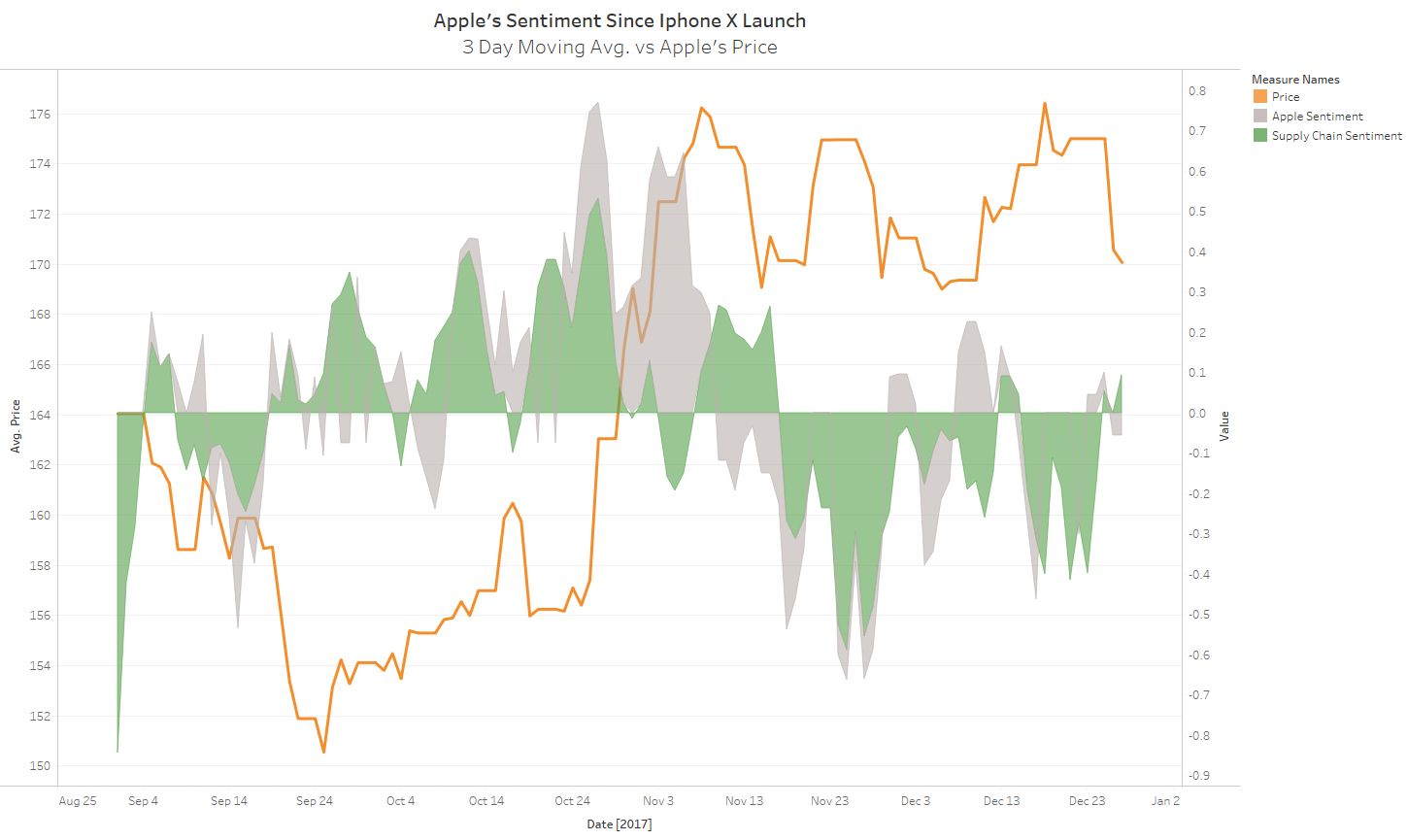
It is no secret that news moves markets. In today’s information heavy world, investors who can more rapidly process and act on events affecting their portfolios will maintain a competitive edge. Alexandria’s global coverage and quality content provide investors with insights to compliment both quantitative and fundamental workflows.
If you have any questions or would like to learn more about any of the content mentioned above please contact us at sales@factset.com.
Please visit the product page on the Open:FactSet Marketplace for more information: Alexandria Contextual Text Analytics.