The Basics
Using pure machine learning, Alexandria has constructed an AI analyst that thinks like a professional but performs like a machine. Its high-performance engine scans tens of thousands of daily financial news items almost instantly and accurately identifies the hidden sentiment, critical facts, and key relationships buried within them. Alexandria Text Analytics – Commodity is generated from highly respected sources covering global macro economies and provides sentiment analysis for commodity-related news, incorporating Alexandria’s event-specific topic models. This gives firms the ability to plug news into investment strategies and gain insight into sentiment on over 45 commodities.
The product information and content statistics contained in this document are as of May 2019.
The Coverage
The Alexandria Text Analytics – Commodity Data Feed provides sentiment for commodity-related new articles covering over 45 commodities. When applicable, articles are tagged with commodity-specific event tags to track sentiment related to shipments, production, inventory and derivative pricing.
Figure 1 below represents the number of articles published per source each year. The number of articles analyzed by Alexandria mirrors the output from various Dow Jones sources. For example, the decline in stories starting in 2009 reflects a concerted effort on the part of Dow Jones to refine its reporting with an increased emphasis on quality over quantity.
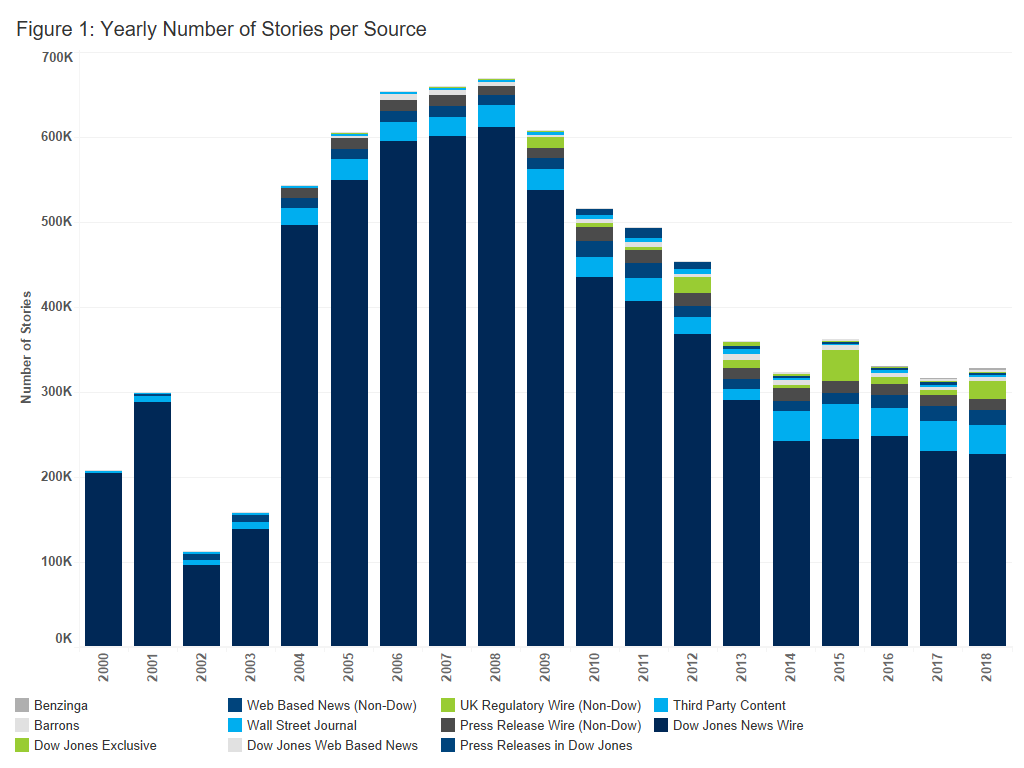
Figure 2 displays the number of stories covering each commodity as of May 2019. The commodity-specific event types assigned to articles include Derivative Pricing, Production, Shipments and Inventory. Figure 3 breaks down the number of stories tagged with each event type for the top five commodities as of May 2019.
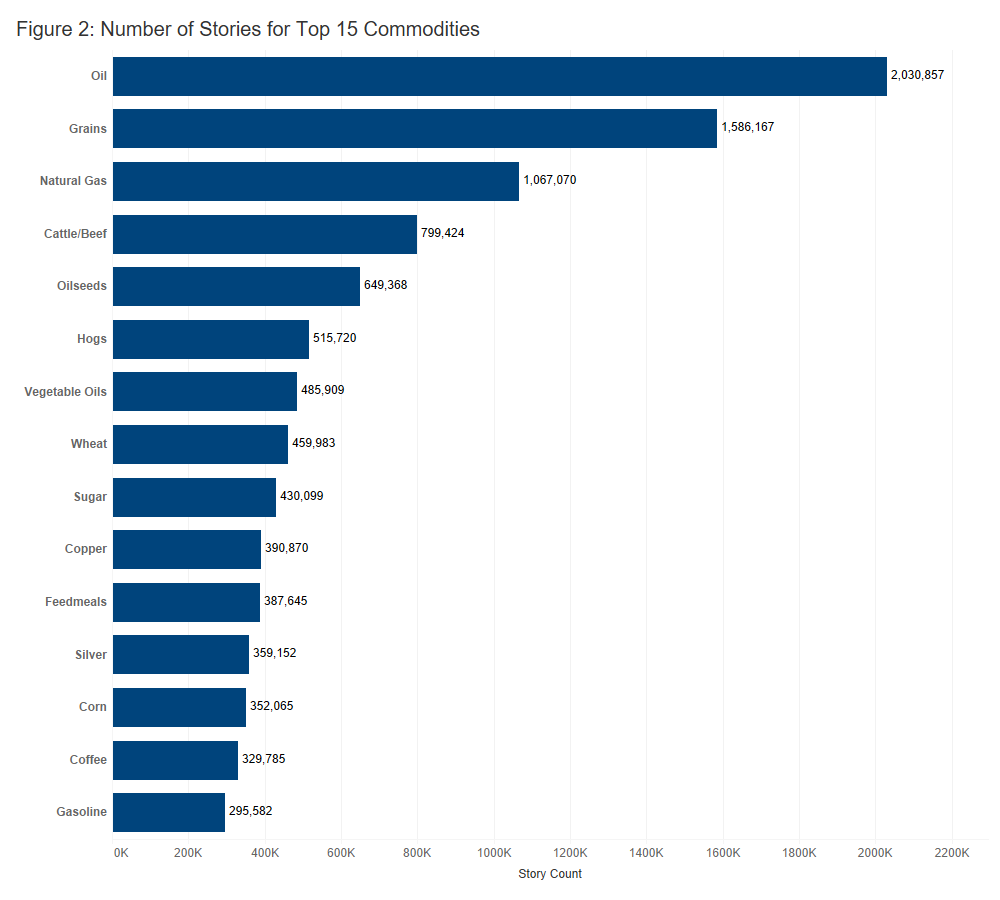

The Differentiators
Beyond coverage and history, key factors to consider when evaluating a news sentiment provider are the content analysis method, sources, classification/event tagging, and entity identification.
Alexandria utilizes artificial intelligence algorithms to analyze sentiment, identify entities, and classify stories by event type. The algorithms are trained using historical news stories reviewed and annotated by domain experts, including buy-side research analysts, Strategists, and CFA charter holders. The Alexandria history dates back to 2000 and has been out of sample since 2005.
Research shows that an artificial intelligence or machine-learning based approach increases the accuracy of event classification and results in a higher rate of non-neutral stories compared to a dictionary or word-list approach. Alexandria’s research also shows that its machine-learning approach matches human-generated classifications over 90% of the time.
The Alexandria commodity coverage includes over 45 commodities, across metals, energy, livestock and meat, and agricultural goods. In addition to a trinary sentiment score, the data set includes a flag for stories that are headlines only, a novelty count per story, as well as metrics around confidence and the probability of a story being positive or negative. All of these can be used to create more nuanced signals from the Alexandria data, such as net sentiment factors based on articles with a high confidence score that are from an institutional news wire source.
Example Use Case
Alexandria Text Analytics – Commodities Data Feed gives users the ability to monitor the sentiment for a variety of commodities. Event tags allow users to customize their analysis by drilling down to news specifically related to derivative pricing, shipping, production or inventory to anticipate the impacts of changing supply and demand dynamics on a global scale. This information adds a powerful layer to commodity trading strategies and traditional factor models capturing macro-economic factors.
This data can be used to predict commodity price movements through examining the market’s response to news sentiment over time. Anticipating commodity prices is not only useful for futures traders, this analysis can be used stay one step ahead of price changes that may impact a company’s supply chain and costs.
Figure 4 illustrates how lumber’s net sentiment over the last three years has mirrored the rise and subsequent collapse of lumber prices. Through 2017 and the beginning of 2018, lumber prices on the Chicago Mercantile Exchange were on the rise in response to a revived U.S. housing market, tariffs on Canadian imports, and reduced lumber supply due to wildfires and distribution difficulties. Over this time, the net sentiment for Forest Products was positive for 19 of 24 months. Net sentiment abruptly turned negative in May 2018 as the price of lumber started to deteriorate and construction and remodeling of homes in the U.S. slowed. While this view of net sentiment is useful to predict lumber prices, it can also be used to anticipate the reaction of lumber mill owners, the strength of the housing market, and improved cost margins for companies producing paper goods.

Alexandria also uses consistent story ids between the Alexandria Text Analytics – Economic, Alexandria Text Analytics – Equity and Alexandria Text Analytics – Commodity Data Feeds, so users with access to multiple data feeds can link an article’s commodity sentiment to the respective economy or company sentiment to further hone analysis.
If you have any questions, please contact us at sales@factset.com.
Please visit the Alexandria Text Analytics – Commodity product page on the Open:FactSet Marketplace for more information.