Alibaba is expected to IPO on August 8th and is positioned to be one of the largest IPOs in history. Due to the size of the IPO the company has received a tremendous amount of attention and scrutiny leading up to its public offering as potential investors strive to place a value on this tech giant. In addition to determining the company’s value, investors will also be interested in understanding the potential impact this company may have on their portfolios. Of course one of the most common ways to examine a security’s impact on a portfolio is to use a factor based risk model.
This got me thinking about the practical implications of adding coverage for new IPOs to risk models. The creators of risk models do their best to cover every relevant security in a market, but inevitably new securities show up all the time and require the means to get these new issues added to the risk model universe. Due to the lack of history available for these securities, it is important to have an understanding of the methods used to add coverage as it could have an impact on portfolios constructed using a risk model.
Consider an ETF that attempts to replicate a benchmark using a small number of securities, an optimizer, and a low target tracking error. If you know how the underlying risk model handles new securities then you can roughly anticipate what your portfolio will need to do in order to maintain its active risk levels. Since FactSet carries models from APT, Axioma, Barra, Northfield, and R-Squared I thought I would run through some of the practices we see from the professionals.
To include or not to include?
First things first, just because a company issues common stock does not mean that it will automatically get included in a risk model, it needs to pass some filters first. Most risk models use criteria like minimum market capitalization or index membership to make this determination. Since many expect Alibaba to raise between $15-$20 billion at IPO (indicating a total value in the vicinity of $150+ billion) and it is a likely candidate for early inclusion into major benchmark families, I think we can safely assume that it will pass most risk models screening rules.
So the security has made the cut, what's next?
Once the decision has been made to include the IPO in a risk model it is necessary to ask the question how? By definition there is no historical returns data available for new securities so model builders need to come up with a methodology to profile a security. This will allow them to estimate the factor exposures and stock specific risk for the new asset. The details of how this is exactly handled vary between major risk vendors but they all do something relatively similar.
So, how do you generate a profile if you don’t have enough historical returns? You can either wait until you do have sufficient history or you use something to act as a proxy. There is a lot of information available the moment a security IPOs such as market cap, country, currency, and sector. This information gives risk model builders some options: they could use some kind of logic to identify similar securities and then derive peer group average factor exposures and residuals which can be used for the new security; similarly they could generate hypothetical synthetic historic returns for the new security, which could then be used to calculate factor exposures and a unique stock specific risk; or, as mentioned above, they could just wait until there is enough history for it to naturally be included in the model. If they do choose to use a proxy approach, then a security can be included quickly into a model and exposures weighted so that over time risk will be derived to a greater proportion by the new security’s realized returns.
How soon is soon?
Assuming that a security can and should be included in a risk model universe, the final question is when should you expect that to actually happen? In the case of the models that are available on FactSet, it depends partly on their frequency and the approach used to add coverage. Those models that have a daily update frequency and proxy the initial risk will include newly covered securities by the end of the IPO date. In the case of weekly models that rely on actual returns data, we can expect these to show up two to three weeks after the IPO. In the case of monthly models, if the minimum data required for coverage is available prior to the month-end then the security will be included in the month-end update.
So what?
After all of that work determining whether or not a security should be covered by a model and then actually generating exposures, one question remains: Does adding coverage early really matter (especially in the short term)? Let’s see what happened when Facebook (FB-USA) IPO’d in May 2012, which was another large and well publicized IPO.
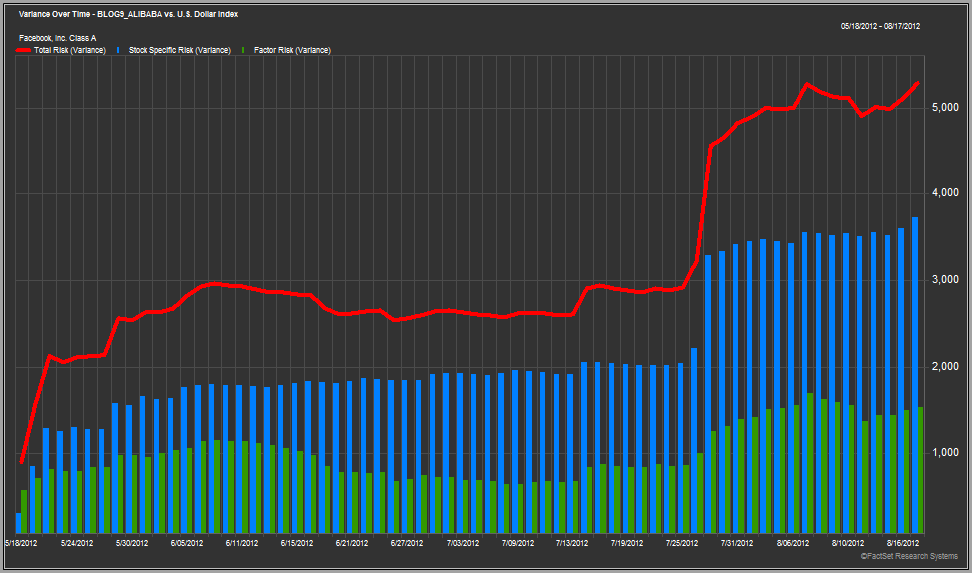
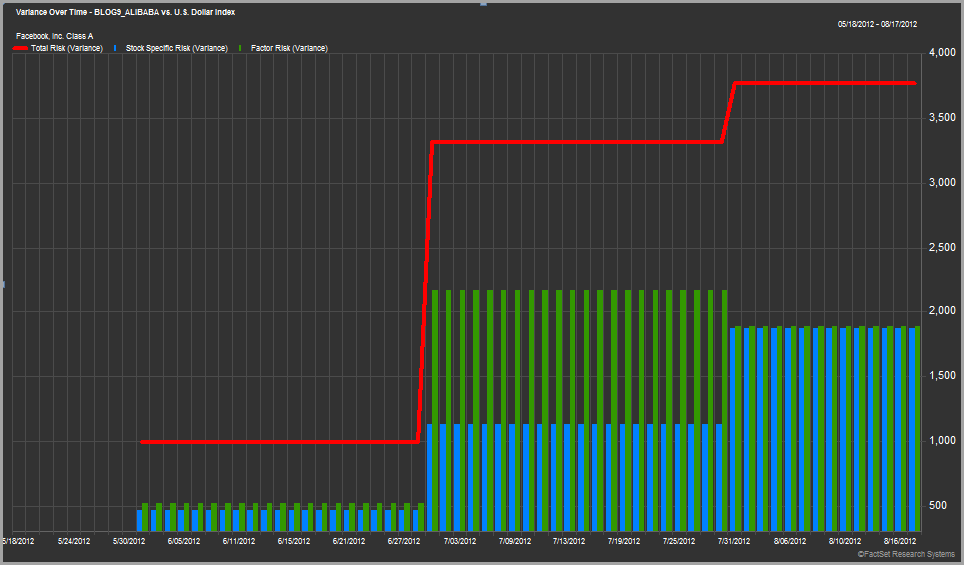
The charts above show the total variance of a single stock portfolio holding just Facebook for the first three months after its initial public offering. The red line represents the total risk, the blue bars represent the stock specific risk, and the green bars the factor risk. The risk estimates are calculated using two different global risk models. The top model is daily and bottom one is monthly, which nicely demonstrates how the different models react over short horizons.
This is far from a conclusive analysis, but what I found interesting is that both risk models show a rapid increase in Facebook’s total risk shortly after the IPO. The first model shows a significant rise in the stock specific portion whereas the second model shows a big jump in the factor risk. This leads to many potential questions: Does this tell us anything meaningful? Why did they both under estimate risk by so much? Do they always get it wrong?
Before we jump to any conclusions let’s look at another example. Below are the same charts for the General Motors (GM-USA) IPO in November 2010.
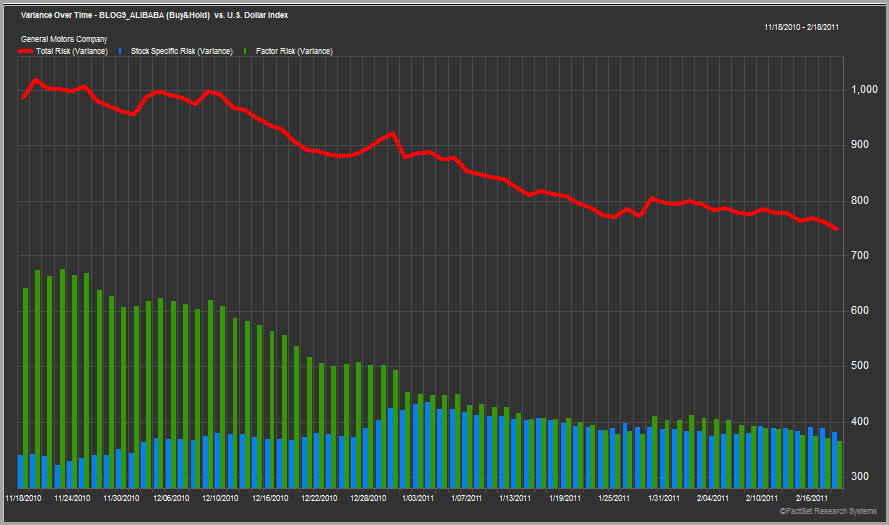
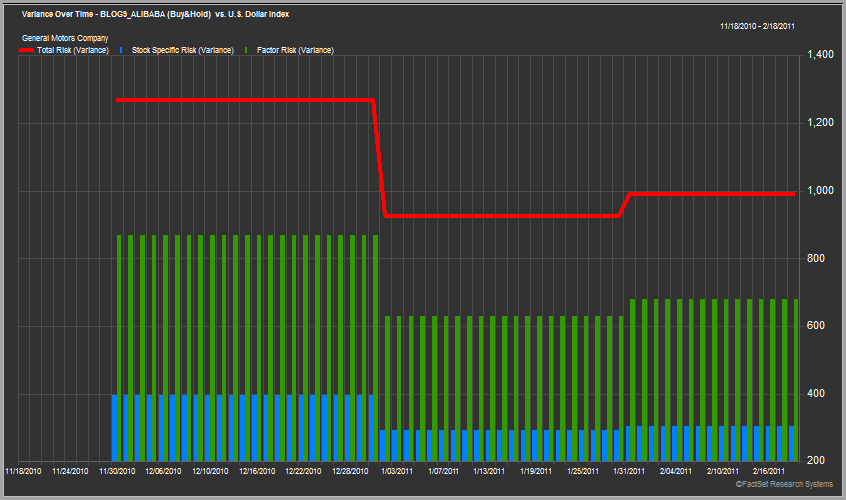
The results are rather different for GM. You could argue that both models slightly over estimated GM’s risk in the beginning, but the changes were relatively moderate and gradual (especially when compared to the Facebook example). To me it looks like the models both did a pretty good job of estimating the risk of this security.
Two separate IPOs and two different results – what does that tell us? Well to me it simply confirms what we already know and need to remember about risk models and that is they are just that – "models”. Specifically they are models that attempt to estimate a security’s risk based on historic market data. In the case of an IPO the normal data used as an input to the model does not yet exist, so there is every chance that the estimates they make will miss the mark and change significantly as data does become available.
The difference we see with the two IPOs above is actually somewhat intuitive. Prior to the 2010 IPO, GM had a long history as a public company. Even when it was bailed out by the U.S. Government there was no shortage of publicly available data, so the IPO price accurately reflected market information and sentiment. Also, risk models had a lot of information about the auto industry in the U.S. to use when estimating risk of such a company, so it makes sense that the initial risk estimates were pretty accurate. On the other hand, Facebook was a very different scenario. There was certainly a lot of public interest in the company and its IPO, but Facebook was a private company with no significant peers to be compared to. The lack of data not only made it difficult to set an appropriate IPO price, but also made it a challenge to estimate risk.
One last chart that seems support the idea that the models themselves are only as good as the data used to build them is below. The chart shows the daily returns of Facebook (grey) and General Motors (light blue) over their first 100 days of trading. What we see is that the price of Facebook was much more volatile (especially in the first 15-20 days) compared to GM. That return volatility suggests that there was probably a lot less certainty about the business of a new giant internet company operating in the relatively new social media space, so the risk models understandably underestimated the stocks risk initially. GM on the other hand was much more of a known quantity: it’s a company that has been around forever in an industry that has been around forever, so the initial risk estimated by the models was pretty accurate.
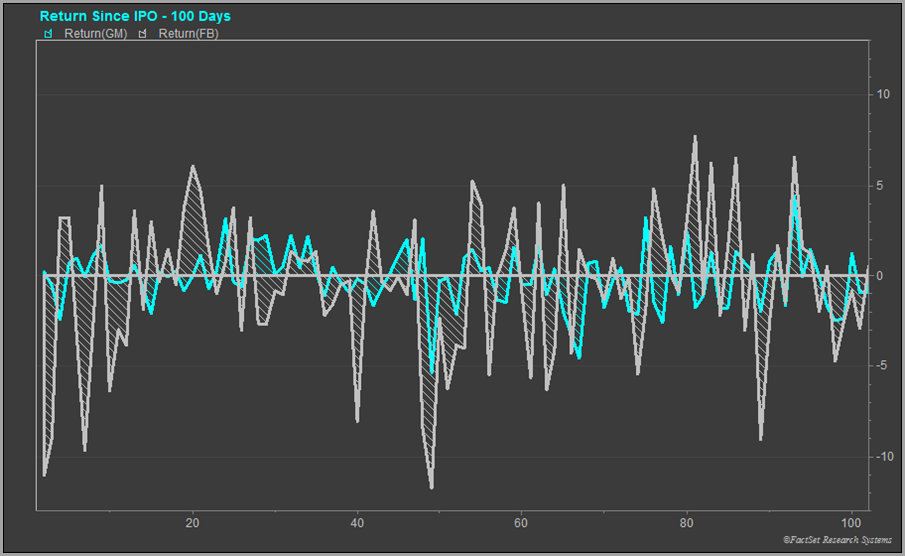
So does adding coverage for new securities early really matter? I guess it ultimately depends on your perspective. If you manage a full replicating index fund then you will likely mimic what the benchmark holds regardless of whether or not a risk vendor covers a security, so coverage is somewhat moot. On the other hand, if you manage a relatively active strategy that attempts to make bets on particular factors then having a significant, new security covered has the potential to alter your decisions and holdings, so having coverage could be much more important. As we saw above, models sometimes get it right and sometimes they don’t. At a minimum you should work to understand the methodology employed by a risk model so that you can try to anticipate the impact and are not completely surprised by the results.
Will the risk models do a good job in estimating the risk of Alibaba when it IPOs in the coming weeks? I won’t hazard a guess, but I certainly think there are some significant informational gaps that will pose problems for the underwriters and the model vendors, so it will be interesting to see what happens.
If you are interested in understanding the exact mechanics of how different providers handle IPOs each vendor is well-equipped to provide further details.